Exploring Challenges and Opportunities to Support Designers in Learning to Co-create with AI-based Manufacturing Design Tools
Carnegie Mellon University
Abstract
AI-based design tools are proliferating in professional software to assist engineering and industrial designers in complex manufacturing and design tasks. These tools take on more agentic roles than traditional computer-aided design tools and are often portrayed as âco-creators.â Yet, working effectively with such systems requires different skills than working with complex CAD tools alone. To date, we know little about how engineering designers learn to work with AI-based design tools. In this study, we observed trained designers as they learned to work with two AI-based tools on a realistic design task. We find that designers face many challenges in learning to effectively co-create with current systems, including challenges in understanding and adjusting AI outputs and in communicating their design goals. Based on our findings, we highlight several design opportunities to better support designer-AI co-creation.
Research Questions
RQ1a | What challenges do designers face when learning to co-create with computational AI tools? |
RQ1b | How do designers overcome these challenges? |
RQ2 | What are effective strategies to support designers in learning to co-create with computational AI tools? |
RQ3 | What are designersâ needs and expectations for human-AI co-creation? |
Method
We conducted a series of think-aloud studies and interviews with 18 trained designers new to working with AI, where they worked with one of two AI design tools (Autodesk Fusion 360 Generative Design and SimuLearn) to complete a realistic advanced manufacturing design challenge.
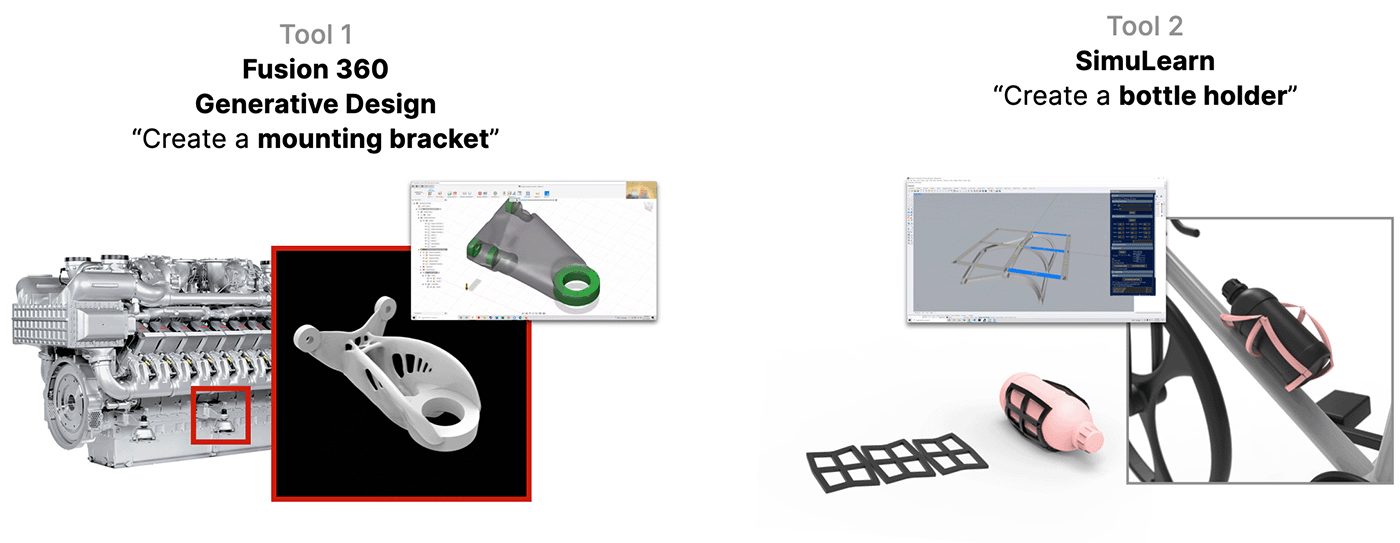
Study 1: Procedure
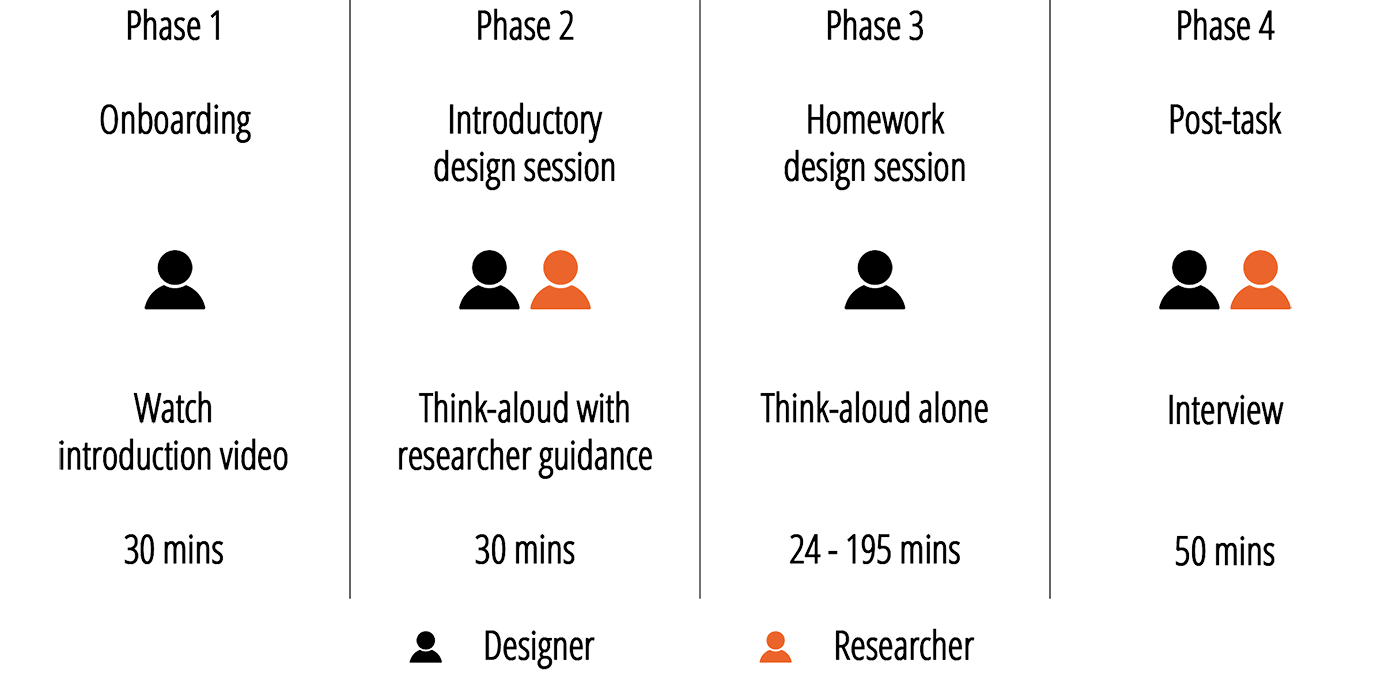
Overview of Study 1 think-aloud design sessions procedure. Participants were frst introduced to the design tool and task, then worked while thinking aloud across multiple sessions. They completed an interview after turning in their design.
Study 1: Collected Data
We collected over 40 hours of video and transcripts of think-aloud design sessions which we analyzed using video interaction and thematic analysis.
- Video and audio recordings and machine-generated transcripts of the open-ended think-aloud design sessions
- Video and audio recordings and machine-generated transcripts of the open-ended think-aloud design sessions
- Audio recordings and machine-generated transcripts of the post-task interviews
- 3D designs created during the think-aloud sessions
Study 1: Findings
Overall, participants expressed seeing potential value in the AI systems to support their design processâespecially that the tools would enable them (at least in theory) to create and explore more complex designs in a shorter time than without AI. However, most faced unresolvable challenges in learning to effectively co-create with the tools.
Design Outcomes: Engine Bracket Task
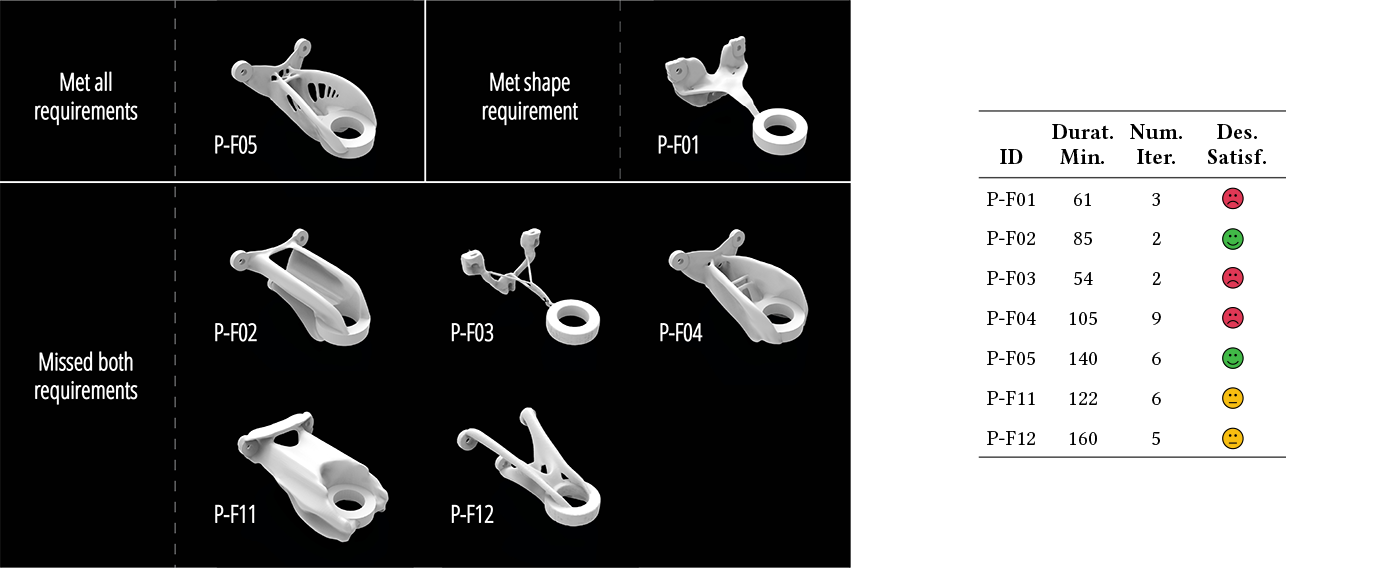
Overview of engine bracket designs created by participants. P-F05 met both the shape and structural requirements. P-F01 only met the shape requirements (the bracket has holes for bolts with sufficient clearance and respects the specified dimensions but is structurally too weak). All other brackets missed both requirements and were either too heavy, weak, larger than the specified dimensions, or had not enough bolt clearance.
Design Outcomes: Bottle Holder Task
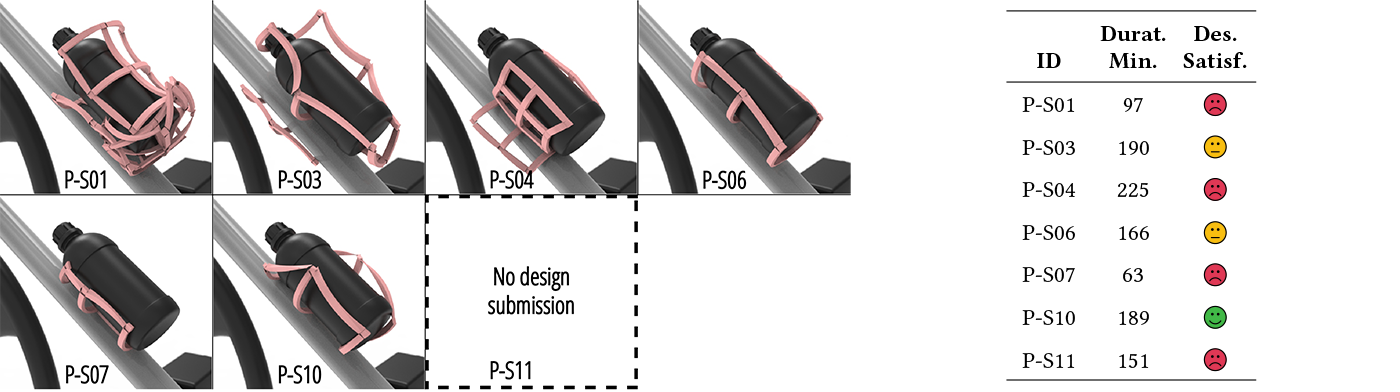
Overview of bottle holder designs created by participants. Participants came up with different designs using the shape-changing grid material in various ways. Participant P-S01 was not able to control the shape as intended and improvised by stacking grids together. While some designs (P-S06, P-S07) would not be able to hold a bottle, some designers (P-S04, P-S10) utilized the shape-changing grid structure to create promising bottle holder designs. Participant P-S11 stopped working on the task out of frustration and did not submit a design.
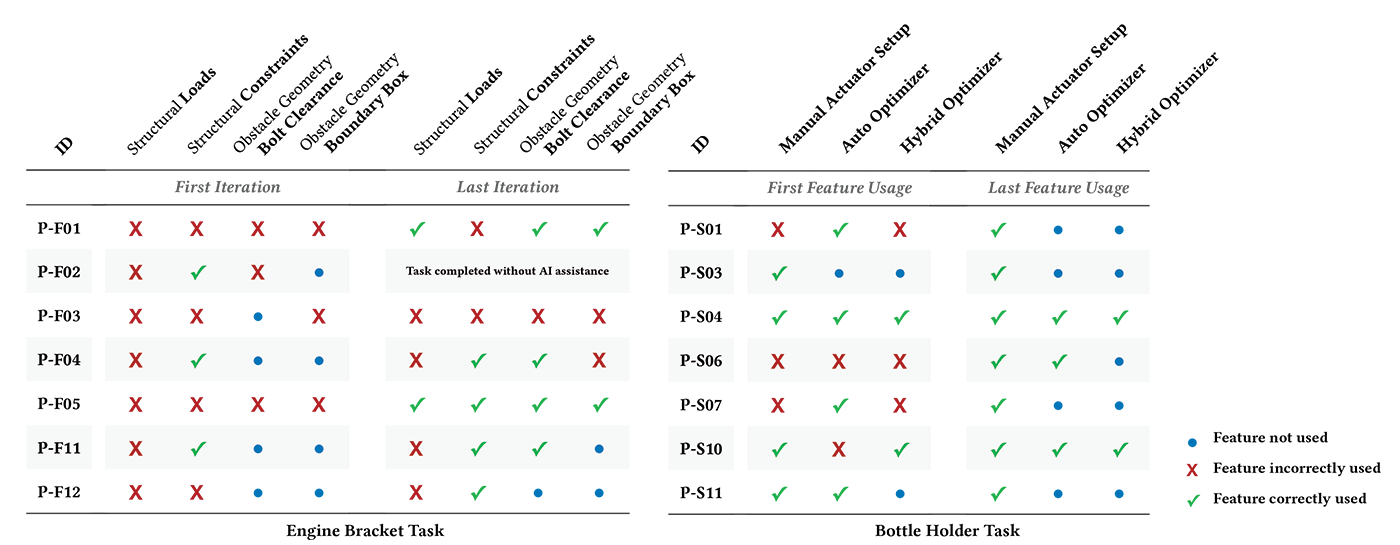
Schematic overview of each participant's learning process of design task-relevant features of the AI system. Left: Engine Bracket Task. Right: Bottle Holder Task. Correct use of input parameters or AI tools is shown with check marks. Increasing numbers of check marks from the first iteration to the last iteration suggest participants learning to work with the AI system.
Challenges designers faced when learning to co-create with AI (RQ1a)
1. Challenges understanding and adjusting AI outputs
2. Challenges working âcollaborativelyâ with the AI
3. Challenges communicating design goals to the AI
Learning strategies of successful designers (RQ1b)
Even though most designers struggled to learn to co-create with the tools, some employed strategies that helped them learn to work better with the AI features.
Systematically exploring AIâs limitations and capabilities
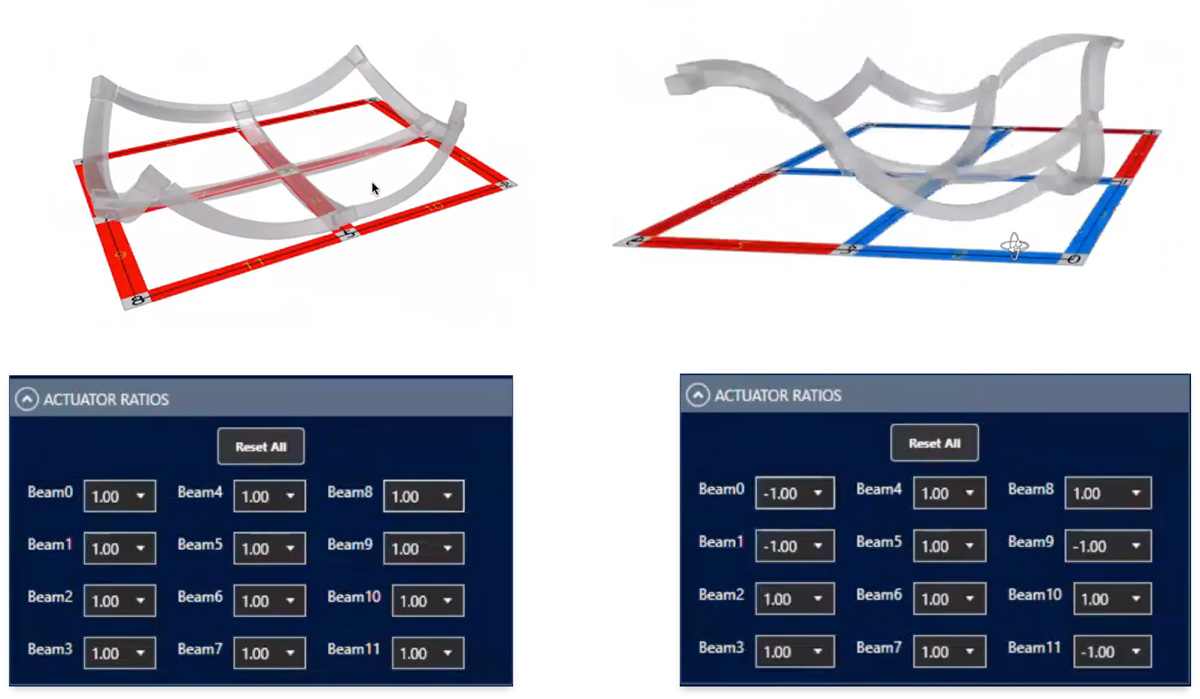
Some designers systematically experimented with the tool to understand better the AIâs behavior, which helped them to refine their mental model by comparing the influence of parameters on the final result.
Sketching, explaining, and reflecting on design issues
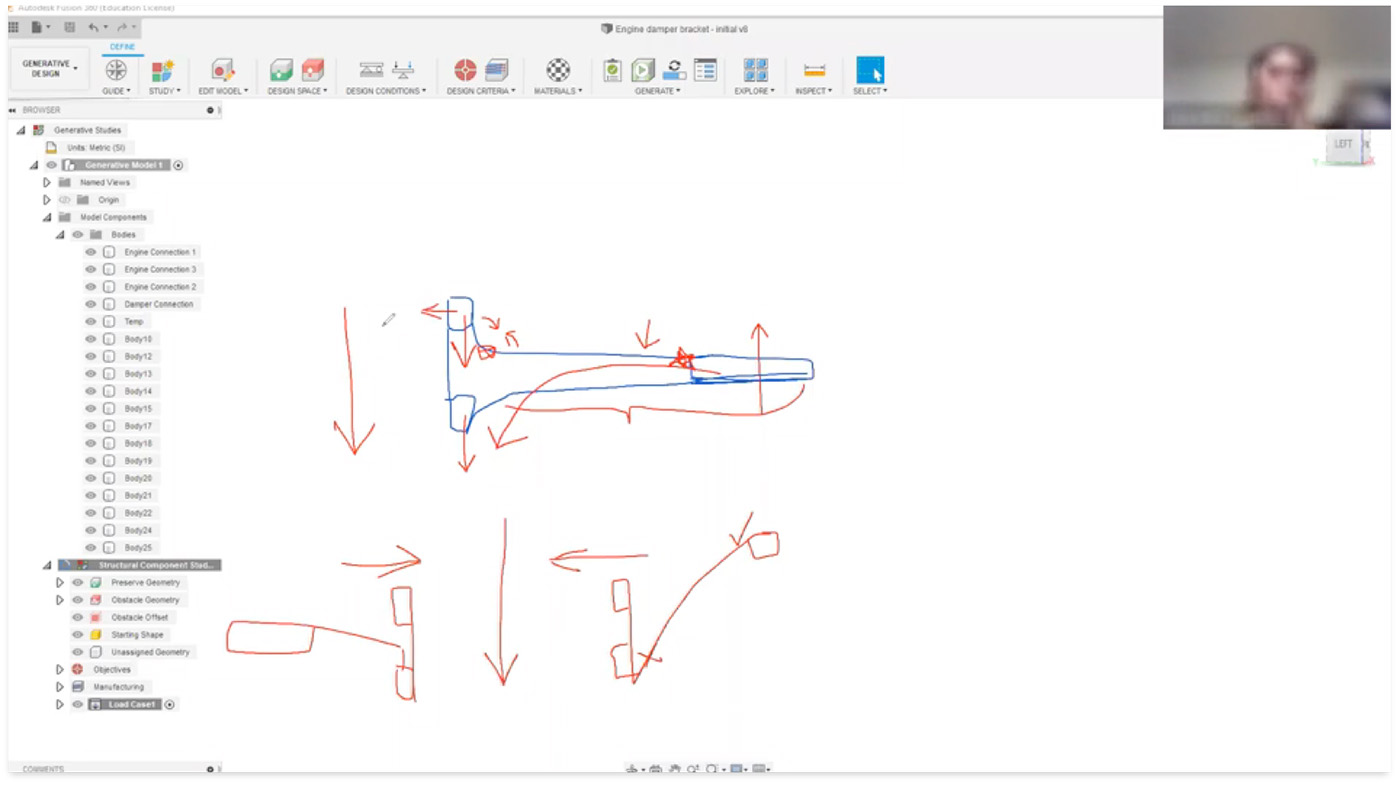
Another strategy was actively abstracting and explaining problems to themselves. For example, when over-constraining the engine bracket, some designers could understand and overcome the issue by sketching out a free-body diagram and explaining the acting forces and constraints to themselves.
Study 2: Learning with a peer guide
To gain insights into how designers can be better supported in learning to co-create with computational AI tools, we conducted additional think-aloud sessions where designers were paired with experienced peers to support them during the task.
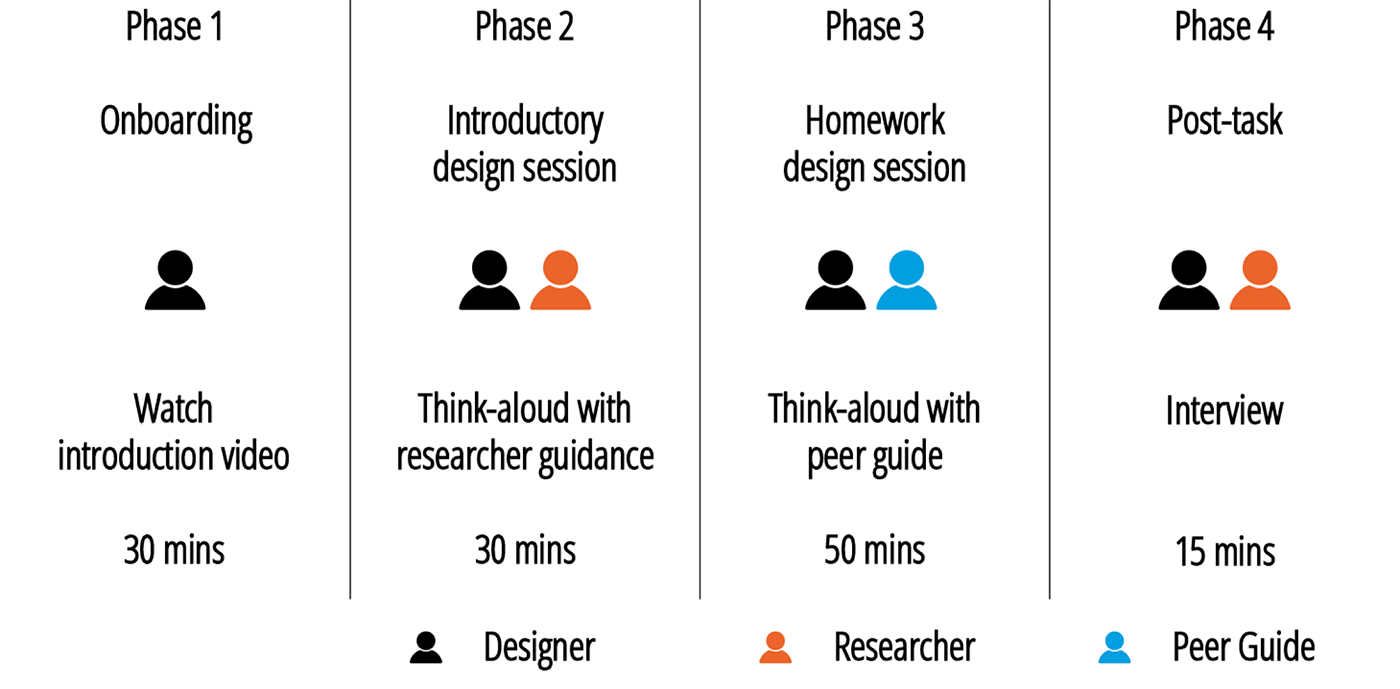
Overview of Study 2 procedure: During the design homework task, designers were remotely accompanied by experienced peer guides.
Study 2: Findings (RQ2)
Successful support strategies of peer guides
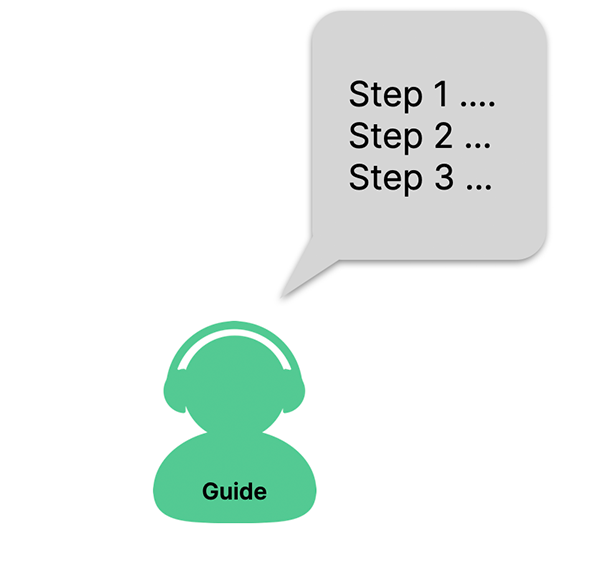
Providing step-by-step walk-through instructions
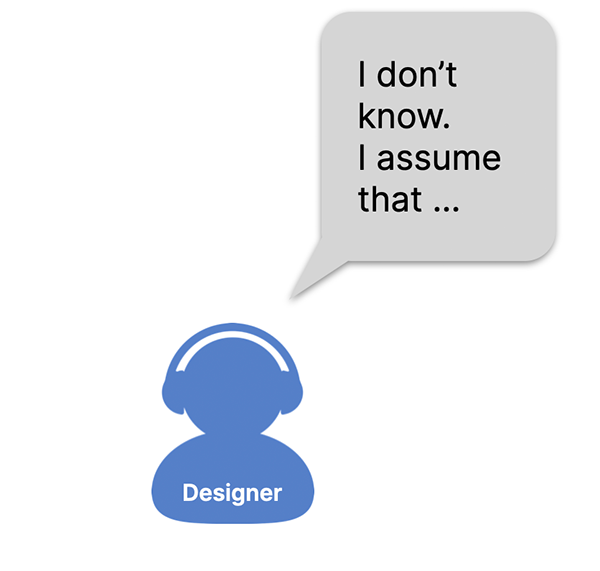
Reacting to designersâ expressions of uncertainty
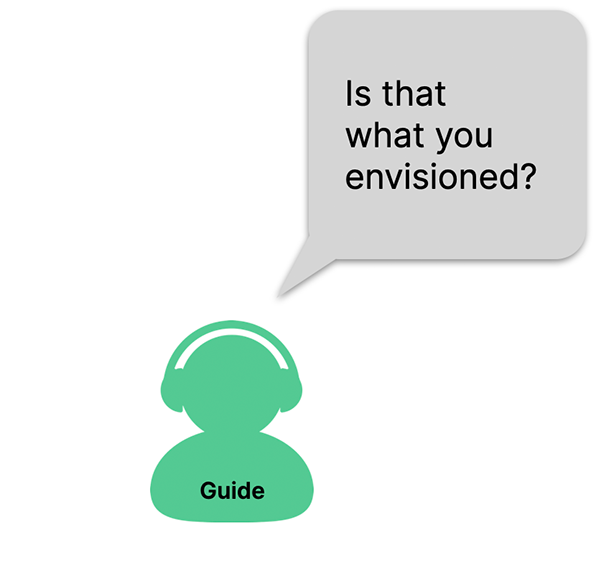
Prompting designersâ reflection on generated designs
Designers Needs and Expectations for Co-creating with AI-based Design Tools (RQ3)
Designers expect from âcollaborativeâ AI-based design tools âŚ
- more contextual awareness of the design problem
- more conversational form of interaction
- support in thinking through design problems
- providing project-relevant work examples
âď¸ Implications
Our findings suggest that the challenges designers faced when learning to co-create with the tools were partly new learning challenges due to the tool's more active role in the design process. We conclude that these new learning challenges also require new support strategies.
Design Opportunities for Future AI-based Design Tools
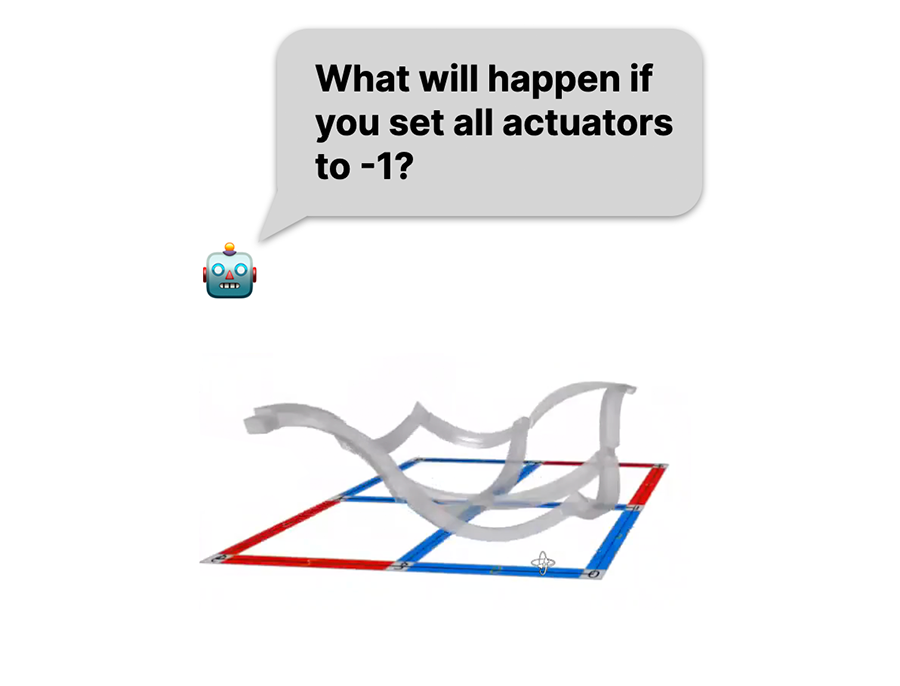
Scaffolding inductive learning of AIâs capabilities and limitations
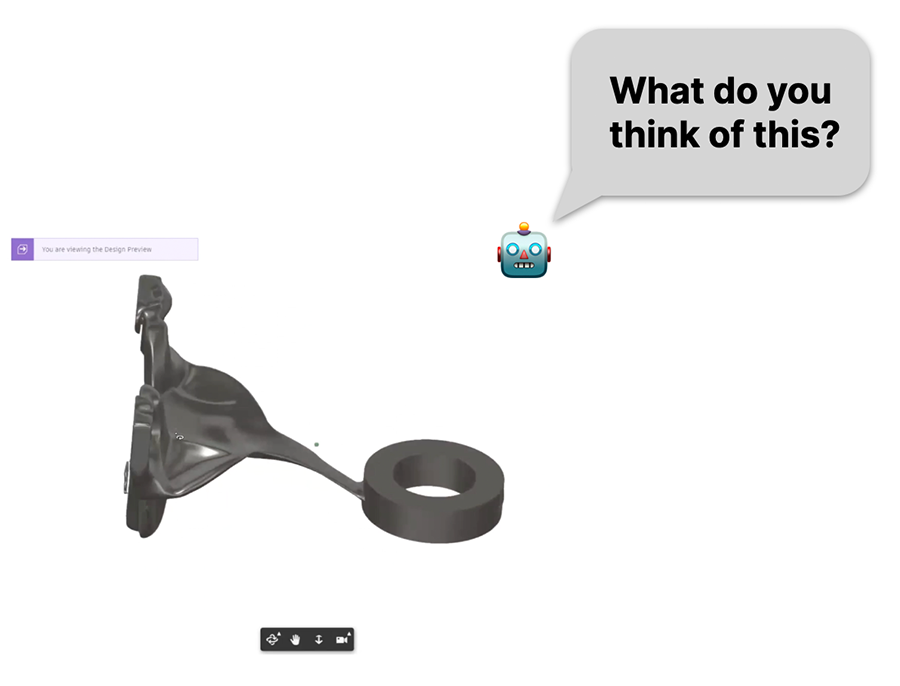
Prompting designersâ planning and reflection
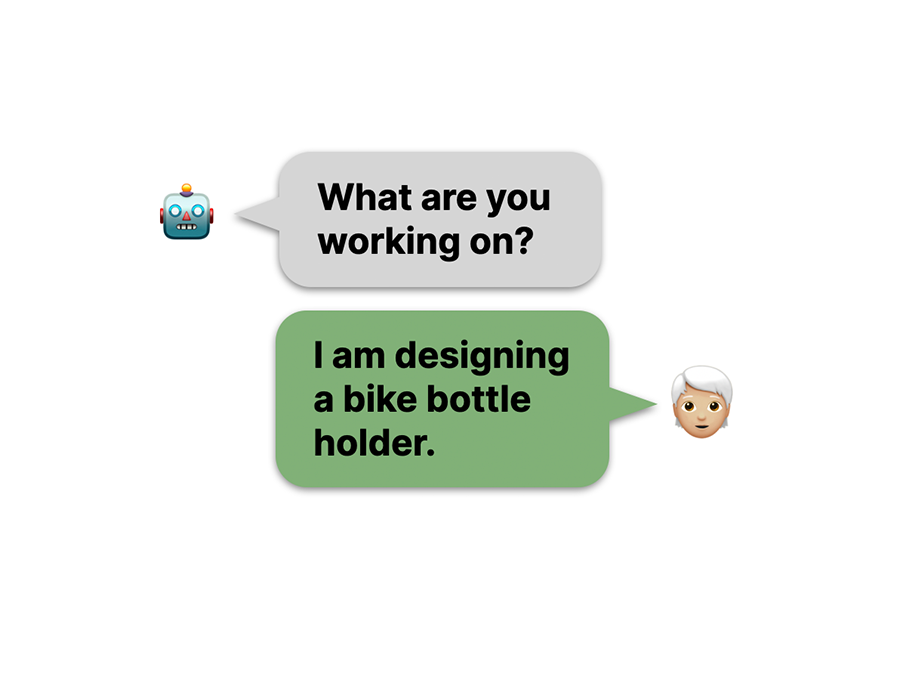
Improving toolsâ contextual awareness of designersâ tasks and objectives
We highlight design opportunities to better support designer-AI co-creation by scaffolding designers in actively exploring the boundaries of AI capabilities and limitations, prompting designers to actively reflect on design problems and observed AI behaviors, enhancing AI systems' contextual awareness of designers' tasks and objectives, and supporting more conversational forms of multi-modal communication between designers and AI systems.